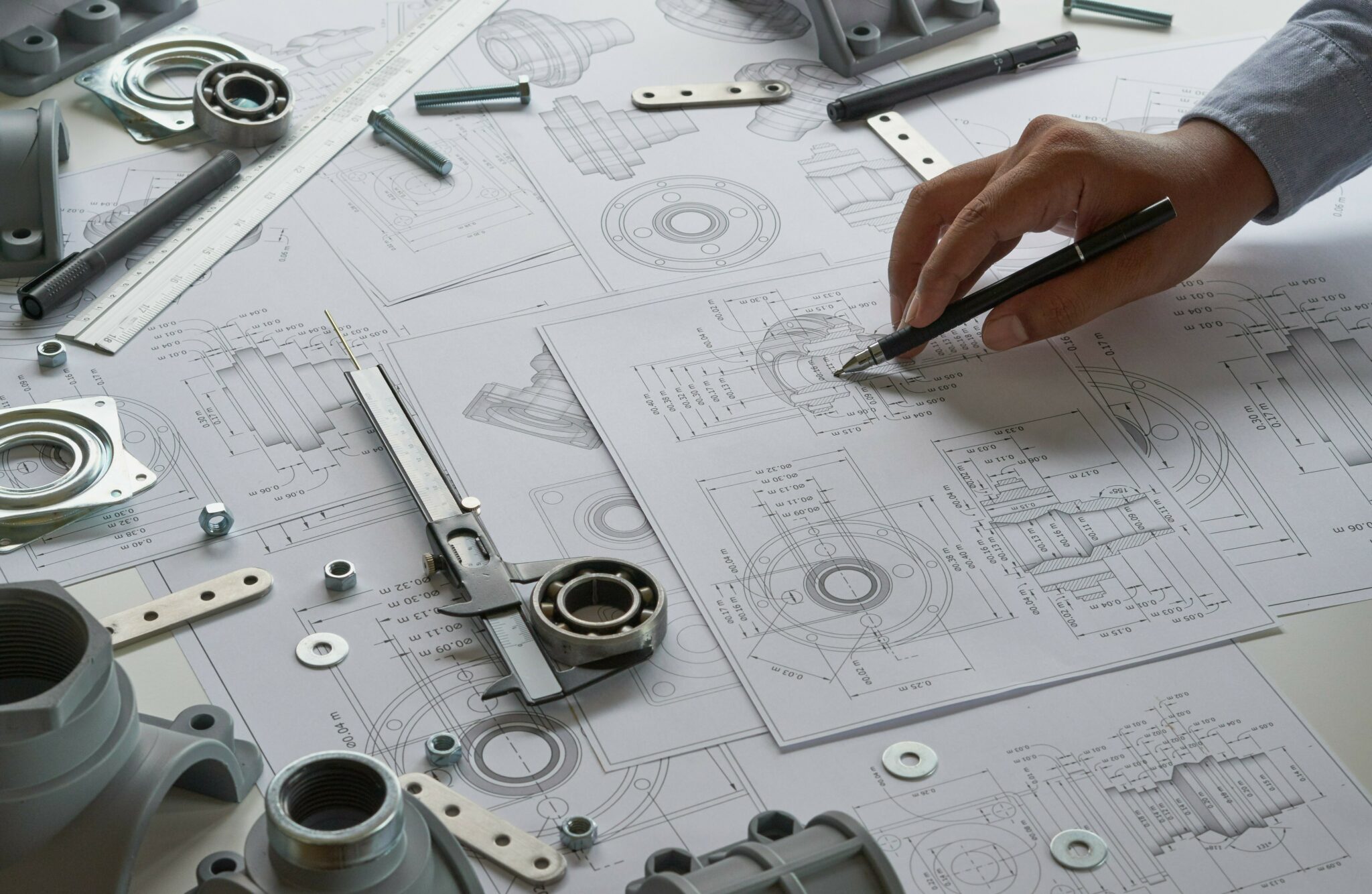
Projects
Our projects are the culmination of industry leading experts & world-class facilities coming together to generate innovative solutions that empower our world with clean energy from start to finish.
Regions
- All Regions
- Canada
- United States
- Germany
- Romania
- United Kingdom
- India
- Korea
- International
Themes
- All Themes
- Innovation
- Sustainability
- Collaboration
- Careers
- Community
- Quality Assurance, Accreditations & Certifications
- Subsidiaries & Partnerships
- Health & Safety
Solutions
- All Solutions
-
Energy & Infrastructure
-
Nuclear Energy
-
Renewable Energy
-
Transmission Support
-
Distribution Support
-
Enabling Technology
-
Hydrogen Energy Systems
-
Hydrogen Energy Systems
-
Regulatory Compliance
-
Fusion
-
Nuclear Energy
-
Electricity Lifecycle
-
New Build
-
Operations & Outage Services
-
Asset Management & Maintenance
-
Modifications & Improvements
-
Decommissioning & Waste Management
-
New Build
-
Components & Systems
-
Electrical Components
-
Nuclear Components
-
Pressure Systems
-
Rotating Equipment
-
Electrical Components
Capabilities
- All Capabilities
-
Facilities
-
Transmission & Distribution Testing Facilities
-
Overhead Conductor & Accessories Testing
-
Connector Labs
-
Fiber Optic Testing & Hardware Services
-
GridSim Lab Dynamic Power Testing
-
High Current Testing
-
High Temperature Low Sag Conductor Testing
-
High Voltage Laboratory
-
Protection & Control Verification
-
Mechanical Testing Lab Services
-
Rotating Machines Testing & Inspection Services
-
Overhead Conductor & Accessories Testing
-
PPE Testing & Laundering Facilities
-
ArcWear PPE Testing
-
Electrical Safety PPE Testing
-
Mask & Surgical Gown Testing
-
Radioactive Laundry
-
PPE Testing & Overhead Line Condition Assessment Facility
-
ArcWear PPE Testing
-
Nuclear Equipment Development Facilities
-
Mock-Ups & Training Facility
-
Nuclear Component Testing Facilities
-
Mock-Ups & Training Facility
-
Nuclear Licensed Facilities
-
Ontario Nuclear Services
-
Radioactive Laboratory
-
Decontamination & Refurbishment
-
Ontario Nuclear Services
-
Materials Laboratories
-
Burst Testing
-
Burst Testing
-
Transmission & Distribution Testing Facilities
-
Services
-
T&D Field Inspection Services & Testing
-
Arc Hazard Assessment
-
Arc Testing
-
Bucket Truck & Live Line Tool Testing
-
Commissioning Engineering
-
Distribution Engineering & Consulting
-
Ground Grid Testing Solutions
-
Overhead Line Inspection
-
Power System Studies
-
Stations & Underground Cables
-
Substation Testing & Commissioning
-
Tower Vibration Monitoring
-
Transformers
-
Transmission Cable Field Testing
-
Off-Shore Array HV Cable System Commissioning Testing
-
High Voltage Direct Current Testing & Commissioning Unit Rental
-
Substation Fencing Solutions – PowerKage™
-
Arc Hazard Assessment
-
PPE Testing & Servicing
-
Synthetic Blood for PPE Testing
-
Synthetic Blood for PPE Testing
-
Nuclear Engineering & Design
-
SMRs, Advanced Reactors & Associated Technologies
-
Cybersecurity
-
Decommissioning Planning
-
Electrical Design
-
Environmental Qualification Engineering
-
Facilities, Infrastructure & Modular Engineering
-
Human Factors
-
I&C and Computer Design Engineering
-
Design Innovations & 3D Modeling Services
-
Mechanical, Civil & Structural Engineering
-
Procurement Engineering
-
Seismic Analysis
-
Stress Analysis
-
Stress-Free Structural Integrity
-
SMRs, Advanced Reactors & Associated Technologies
-
Business & Project Management
-
Automated Workflow Processes & Business Intelligence
-
Project Controls
-
Project Management Support Services
-
Automated Workflow Processes & Business Intelligence
-
Nuclear Licensing & Safety
-
Deterministic Safety Analysis
-
Licensing & Regulatory Compliance
-
Probabilistic Safety Assessment & Risk Assessment
-
Radiation Safety & Shielding
-
Thermal Hydraulics Analysis & Design
-
Nuclear Security & Safeguards
-
Environmental Consulting & Community Engagement
-
Deterministic Safety Analysis
-
Lifecycle Management
-
Advanced Materials & Asset Utilization for Electrical Systems
-
Asset Management Services for Electrical Systems
-
CANDU Fuel Channel Fitness-For-Service Solutions
-
Fitness-For-Service & Advanced Methodologies
-
Lifecycle Management & Component Integrity
-
Life Extension & Station Refurbishment
-
AI & Machine Learning Services
-
Advanced Materials & Asset Utilization for Electrical Systems
-
Nuclear Services, Site & Outage Support
-
Concrete Assessment & Repair
-
Degraded Nuclear Piping
-
Equipment Decontamination, Maintenance & Refurbishment
-
Feeder Inspection
-
Field & Project Engineering
-
Fuel Channel Inspection
-
Health Physics
-
Integrated Source Term Monitoring
-
Filter Performance Testing
-
Outage Activity Transport Monitoring
-
Stack Monitor Commissioning & Testing
-
Steam Generator Inspection
-
Local Liaison, Services & Engineering
-
Concrete Assessment & Repair
-
Nuclear Equipment Development & Supply
-
Process Skid Design & Fabrication
-
Reverse Engineering Services
-
First-of-a-Kind Robotics Development & Testing
-
Tooling Development & Testing
-
Tool Maintenance
-
Process Skid Design & Fabrication
-
Materials & Nuclear Component Testing
-
Commercial Grade Dedication
-
Corrosion Testing
-
Environmental Qualification
-
Expert Materials Consultation
-
Irradiated Material Characterization & Testing
-
Loss of Coolant Accident Testing
-
Machining & Prototyping
-
Nuclear Material Degradation Testing & Research
-
Seismic Testing Equipment
-
Commercial Grade Dedication
-
Forensic & Failure Analysis
-
Distribution Equipment Assessment
-
Field & Asset Inspection
-
Low, Medium & High Voltage Cables
-
Transformer Assessment
-
Distribution Equipment Assessment
-
Chemistry & Assaying
-
Dosimetry Services
-
Analytical & Radioanalytical Chemistry
-
Tritium & Deuterium
-
Dosimetry Services
-
Radioactive Waste Management
-
Radioactive Waste Characterization
-
Radioactive Waste Processing
-
Radioactive Waste Characterization
-
Medical Isotopes
-
Isotope Irradiation
-
Isotope Irradiation
-
T&D Field Inspection Services & Testing
-
Products
-
Health & Safety Products
-
Arc Flash Analysis Software
-
Personal Protective Equipment (PPE) Products
-
Radiological Safety Analysis Software
-
Arc Flash Analysis Software
-
Isotope Products
-
Medical Isotope Products
-
Medical Isotope Products
-
Nuclear Generation Products
-
Feeder Inspection & Maintenance
-
Fuel Channel Inspection & Maintenance
-
Steam Generator Inspection & Maintenance
-
Feeder Inspection & Maintenance
-
Radioactive Waste Management Processes
-
SCEPTER™ Selective Carbon Extraction Process
-
SCEPTER™ Selective Carbon Extraction Process
-
Transmission & Distribution Products
-
Anagen™
-
Anagen™ Freeze
-
LineVue® (Lease Option)
-
Anagen™
-
Health & Safety Products